3394 阅读 2020-02-10 11:22:46 上传
A corpus of read and conversational Austrian German
Barbara Schuppler a,∗ , Martin Hagm¨ullera , Alexander Zahrera
aSignal Processing and Speech Communication Laboratory, Graz University
of Technology, Inffeldgasse 16c, 8010 Graz, Austria
Abstract
This paper presents GRASS (Graz corpus of Read And Spontaneous Speech), the first large scale speech database for Austrian German with both read and conversational speech. In total, the corpus contains approximately 1900 minutes of speech in which 38 speakers produced more than 220 000 word tokens from 14 593 di↵erent word types. The corpus consists of three components. First, the Conversational Speech Component contains free conversations of one hour length between friends, colleagues, couples, or family members. Second, the Commands Component contains commands and keywords which were either read or elicited by pictures. Third, the Read Speech Component contains phonetically balanced sentences and digits. The speech of all components has been recorded at super-wideband quality in a soundproof recording-studio with head-mounted microphones, large-diaphragm microphones, a laryngograph, and with a video camera. The corpus was fully annotated at the orthographic level, and partly also at the segmental, subsegmental and prosodic level. Our analysis of conversational speech characteristics such as overlapping speech, laughter, repetitions, hesitations and the use of colloquial and dialectal words allows us to conclude that the conversational speech material is highly casual in nature. The collected corpus provides conversational material for phoneticians and linguists interested in topics specific for Austrian German (e.g., pronunciation variability, prosody, syntax of spoken Austrian German), and for those studying talk in interaction in general (turn-taking, grounding, entrainment, extra-linguistic factors etc.). Furthermore, it is a valuable resource for speech technologists inter-ested in the development of ASR and dialogue systems for different speaking styles of Austrian German.
Keywords: Austrian German, read speech, conversational speech, automatic transcription, prosodic transcription
1. Introduction
1German is one of the best documented languages. Speech scientists studying spoken German have the choice among several speech corpora which were recorded at su"ciently high quality and which come with transcriptions at least at the orthographic level. The detail in transcription and the number of speakers is highly variable, depending on the field of application. For instance, large corpora of read and spontaneous speech of many speakers were created with the motivation to train and test speech technology tools (e.g., the Verbmobil Corpus (Weilhammer et al., 2002), the Alcohol Language Corpus (Schiel et al., 2008)). Another corpus with less speakers, but which is suitable for both speech technology and detailed phonetic analyses, is the Kiel Corpus of Spontaneous Speech (IPDS, 1997). It was annotated manually with detailed phonetic and prosodic annotations. A rich resource for language variation and change is the German Today corpus (Caren Brinckmann and Berend, 2008), which contains read and spontaneous speech of 525 participants from 160 cities. Finally, also a large number of smaller corpora have been collected for specific applications (e.g., Berlin Map Task Corpus (Sauer and Rasskazova, 2014), BROTHERS (Feiser, 2015)).
For Austrian German, the available annotated speech material is very limited. For instance, the interview material which Moosm¨uller (1998, 2007) used for her acoustic phonetic study on Austrian German vowels contains read speech (72 sentences per speaker) and spontaneous interviews with a linguist (20 min of speech), but only from five speakers. Moreover, the data was not completely orthographically and phonetically transcribed. Similarly, also the material collected for the Styrialects project, which studies the dialects of Styria, is not available for other speech scientists with complete annotation layers (Steiner and Vollmann, 2010), nor are the spontaneous dialogues collected by Muhr (2000), which contains the speech of 12 speakers from Austria, Germany and Switzerland. A corpus which was fully transcribed is the SpeechDat-AT database. It contains telephone speech from many (= 2000) speakers. The spontaneous speech part, however, is restricted to the spontaneous elicitation of single words (Baum et al., 2000). Another speech database for Austrian German is the ADABA database (Muhr, 2008), which is restricted to sentences and single words read by trained speakers. Finally, Austrian speakers have been recorded in projects with the aim of covering all German speaking countries (e.g., German Today (Caren Brinckmann and Berend, 2008), RVG (Burger and Schiel, 1998)). To the best of our knowledge, there is no existing speech database with conversational Austrian German at all. This paper thus presents the first large scale speech database for Austrian German with both read and conversational speech (GRASS: Graz corpus of Read And Spontaneous Speech)2 .
In the last decade, large corpora of conversational speech have been created among others for English (Pitt et al., 2005), Dutch (Ernestus, 2000; Schuppler, 2011) and French (Torreira et al., 2010). These corpora, however, lack read speech of the same speakers from the same region in the same recording condition, which is required in order to draw conclusions about speaking style. As a consequence, when the findings based on these corpora are compared to those from read speech, it can not be excluded that the observed differences are actually due to (1) different speaker characteristics (2) different recording conditions and/or (3) di↵erent annotation methods. Finally, read speech may not only be helpful as a reference in linguistic and phonetic studies but also when building up a speech recognition system, for instance, for the training and/or adaptation of acoustic models.
The Graz corpus of Read And Spontaneous Speech is designed to be suitable for both linguistic and phonetic studies as well as for the development of automatic speech recognition (ASR) and dialogue systems, comprising the following technical and content-related characteristics:
1. High-quality super-wideband recordings which enable the simulation of different acoustic environments by filtering the speech material with different measured room impulse responses.
2. Phonetically balanced sentences and digits from each speaker, as well as read and elicited commands and keywords as needed for certain dialogue-system applications.
3. Su"cient speech material from free conversations in order to model pronunciation variation and spontaneous dialogue phenomena (hesitations, fillers, overlapping speech).
4. High quality orthographic transcriptions which allow the (semi-automatic) generation of further segmental and supra-segmental annotation layers.
This paper is organized as follows. In the next section, we provide a short insight into the characteristics of Austrian German, with a special focus on the role of speaking style. Then in Section 2, we describe the data collection of the corpus (i.e., the speaker characteristics, the equipment and the recording procedure). Section 3 presents details of the creation of the orthographic, phonetic and prosodic transcriptions. An analysis of conversational speech characteristics such as overlapping speech, laughter, disfluencies is presented in Section 4). Finally, we provide information about the corpus availability.
1.1. Speaking style and regional varieties in Austria
Each of the German speaking countries have their own written and spoken standard language (e.g., standard Swiss and Austrian German). The standard languages partly differ with respect to their lexicons and grammatical structures such as rules for the use of past tenses, articles and cases (D¨urrscheid and Elspaß, 2015). This kind of variation is determined by the country a speaker lives in. The dialectal borders, however, do not match the national borders. For instance, whereas the intonation and pronunciation of a speaker from Vorarlberg (province in Austria at the border to Switzerland) and one from Switzerland are very similar, the lexicon and grammatical structures used may be different in certain aspects.
What speakers from all German speaking regions have in common is that they are capable of producing a broad range of speaking styles. In read speech, the difference between speakers from northern Germany, Switzerland and from Austria remains audible as an accent, but can be assumed not to hinder communication. Similarly, an ASR system trained on German read speech, performs nearly equally good on sentences read by Austrian speakers (Adda-Decker et al., 2013). Some of the most salient pronunciation differences of the standard language in Austria and the standard language in Germany are the devoicing of consonants (i.e., of the voiced plosives and the fricative /s/) and the deaspiration of voiceless plosives (Moosm¨uller and Dressler, 1988; Moosm¨uler and Ringen, 2004; Klaaß, 2008). Furthermore, vowels which are short in standard German may be long in the Austrian varieties, and depending on the region, monophthongations are frequent (Moosm¨uller, 1997; Vollmann and Moosm¨uller, 2001).
In spontaneous conversations, Austrian speakers will switch between different styles, depending on the formality of the situation and the regional background of the interlocutor. For instance, speakers from Vorarlberg (i.e., a province in the west of Austria belonging to the Alemannic dialectal area), speak their regional variety in a conversation with someone from the same area. Since the dialect is hardly comprehensible for a speaker from outside that area, speakers mostly switch to a pronunciation and to a lexicon closer to the standard language in a casual conversation with someone from another region. In formal situations, speakers will switch to the standard language, which may come with a slow speechrate and hyperarticulation.
In addition to the regional background of the speakers, there is variation due to factors such as city size, age and education (Auer et al., 2008). These sociological factors are especially relevant in the cities (William, 2001). To give an example: The pronunciation of a 20-year-old university student from Graz city is more similar to the one of a 25-year-old student from Vienna and Salzburg than to a 25-year-old waitress from a small village of the district around Graz (example on basis of the collected GRASS corpus). Furthermore, due to the influence of media, certain aspects of a dialect may spread over the whole country, (e.g., the monophthongation of /au/ and the vocalization of /l/, both typical phonological processes of Vienna, can now also be found in speakers of the rest of Austria (Vollmann and Moosm¨uller, 2001). Lexical expressions typical for German speakers such as Tsch¨uss ‘bye bye’ and krass ‘incredible!’ can also be found in the speech of young speakers of Austria (example on the basis of the collected GRASS corpus). For a more detailed literature review on the interplay of di↵erent factors on the varieties and the style-continuum spoken in Austria see Hobel and Vollmann (2015).
To sum up, linguistic studies on the variation in Austrian German show that the regional background of a speaker is only one factor for pronunciation variation and that speaking style plays an important role. With the creation of the GRASS corpus, we aim at providing the first resource on conversational speech, which hopefully will be interesting for (1) speech technologists and (2) linguists: (1) Traditionally, input data for an ASR system is separated into planned (e.g., read) and spontaneous, where spontaneous refers to everything which is not scripted (e.g., also interviews). For Austrian German, however, the formal (spontaneous) speaking styles may be closer to read speech than to conversational speech. Therefore, researchers interested in, for instance, building systems doing meeting documentation or in systems for medical operation documentation will profit from the GRASS corpus. (2) So far, most linguistic and phonetic studies investigating the characteristics of Austrian German were based on controlled experiments, read speech or on interviews with a linguist (e.g., Moosm¨uller (2007); Steiner and Vollmann (2010); Leykum et al. (2015)). The GRASS corpus provides conversational material for linguists interested in topics specific for Austrian German (e.g., pronunciation variability, prosody, syntax of spoken Austrian German), and for those studying talk in interaction in general (turn-taking, grounding, entrainment, extra-linguistic factors etc.).
2. Data Collection
Before collecting the data, we carried out a pilot with two speakers to test the equipment, the recording procedure, and the resulting recording quality. After having applied the necessary modifications, we recorded 38 speakers within two weeks with the here presented final set-up and procedure.
2.1. Speakers
The GRASS corpus contains speech produced by 38 speakers (balanced male and female, between 20 and 60 years old). They are moderately educated (at least high school diploma, half of them have a Master degree or higher). We chose to restrict the regional background of the speakers, as we were mainly interested in having a database that allows to investigate the difference between di↵erent speaking styles, rather than differences between the two main dialectal areas, Bavarian (central and southern) and Alemannic. Since our department is situated within the Bavarian dialect zone and since this is also by far the bigger dialectal zone, we decided to exclude speakers with an Alemannic dialect. Table 1 shows the distribution of the regions where they spent their childhood. All speakers worked in Graz at the time of the recordings. Only 9 of the 38 speakers spent their childhood in one of the main cities, all others grew up in smaller towns and villages in Austria (i.e., less than 120 000 inhabitants). To sum up, the speakers are originally mostly of small villages of Austria and have a high level of education.
Table 1 provides an overview of speaker characteristics (age, gender, regional background, education, foreign languages). In addition to the characteristics mentioned in Table 1, we documented information about the speakers’ size, the educational and regional background of their parents, the working area (e.g, technology, social, languages) of the speakers and their parents, their musical education, and whether they received some sort of professional pronunciation training.
Table 1: Information about the speakers. ‘Years of Education’ refers to the years after the obligatory secondary education. ‘L1’ stands for mother tongue. In ‘Foreign Languages’: two stands for two foreign languages and more for more than two foreign languages learned by the speaker.
For the conversational speech, 19 pairs of speakers were recorded. There were both mixed pairs and gender-homogeneous pairs (6 between men, 6 between women and 7 mixed). All conversations were between pairs of speakers who have known each other for several years and they were either colleagues from work (3 conversations), family members (3), friends (10) or couples (3).
2.2. Equipment and sound quality
Figure 1 shows the setup of the equipment for the Conversational Speech Component (left panel) and for the other components (right panel). We recorded the speech of all speakers in the recording studio of the SPSC Laboratory of the Graz University of Technology with a close talking head-set (AKG HC-577L: i.e., HM1 and HM2 in Figure 1) and a large diaphragm microphone (AKG C414 BXLS: i.e., FM1 and FM2 in Figure 1) with attached pop screen. Additionally, all speakers were recorded with a laryngograph (i.e., a device that measures the impedance of the larnyx, which depends on the contact area of the vocal folds; recordings can be used as ground truth for F0 estimation). Finally, most of the conversations (14 out of 19) were recorded with a video camera (Canon Legria HF M31 HD Camcorder). These recordings might be used in the future for the study of gestures, which is relevant for the development of multi-modal dialogue systems.
The .wav files have the format RIFF (little-endian), mono WAVE audio, uncompressed PCM 16 bit, with a sampling frequency of 48kHz. We acquired at 48 kHz sampling rate and then generated a version at 16 kHz. The average SNR over all speakers over the Read Speech and the Commands Component resulted to be 49.7dB. For the conversational speech, the SNR di↵ered strongly between the different speakers. For the recordings with the head-mounted microphones, the lowest SNR was 35.8dB (a female speaker) and the highest was 52.8dB (a male speaker), with an average of 46.2dB for HM1 and 46.4dB for HM2; For the recordings with the large diaphragm microphone the SNR resulted to be lower in average (31.3dB for FM1 and 35.7dB for FM2), which is due to the speakers movements during the conversation. As expected, the SNR of the recordings with the laryngograph were even lower with an average of 28.4dB over all conversations. For the calculations of the SNR values presented in this section, we followed the approach presented in H¨ansler and Schmidt (2004).
2.3. Recording procedure and corpus contents
We first recorded the conversational speech, then the elicited commands. Only after those (semi-) spontaneous tasks, we recorded the read commands and the read sentences. We chose this order of events for several reasons. First, the pairs of speakers mostly arrived at the same time in the recording studio and often simply continued their conversation–which started on their way to the studio–with no interruption. Such an interruption would have meant a change in topic, but most importantly a switch to a different speech style. Second, this order guaranteed that the elicited commands were not influenced by the reading material. Finally, this order of recordings ensured that speakers knew as little as possible about the purpose of the recordings and about the setup in the recording studio.
2.3.1. The Conversational Speech Component (CSC)
For the recordings of the conversational speech, a small table with provocative pictures concerning the topic ‘Living in Graz’ was placed close to the speakers. Speakers were instructed that they could start their conversation by using these cards if they wanted to, but that in principle, they could talk about whatever topic they like. They were told that the recordings would be transcribed afterwards, but that during the recordings nobody would listen. The two speakers were left without watch nor mobile phone in the recording room for one hour. Only one quarter of the pairs of speakers started with the cards provided. In total, 19 conversations were recorded, each of one hour length, containing a total of 198 129 word tokens from 13 231 di↵erent word types. Section 4 presents an analysis of lexical and pronunciation-related characteristics which show the casualness of the speech recorded.
2.3.2. The Commands Component (CC)
All speakers produced 15 commands and 5 keywords while being presented an image indicating which object inside an apartment shall be operated by a voice controlled system. In total, the recorded elicited commands and keywords contain 1 720 word tokens from 464 word types. Furthermore, speakers read 15 commands of the type Open the window, please! Turn off the light! and 10 keywords of the type Wake up! as used in common voice control system. In total, the read commands and keywords contain 3 853 word tokens from 270 different word types.
2.3.3. The Read Speech Component (RSC)
Each of the 38 speakers read approximately 62 phonetically balanced sentences, which were taken from the Kiel Corpus of Read Speech (IPDS, 1997), and 4 telephone numbers. Additionally, the speakers read 10 utterances with a spontaneous speech like structure (i.e., sentence fragments), containing word tokens which were also expected to occur frequently in the CSC of the corpus. We collected these sentences to be able to draw better comparison with the CSC. In total, the RSC consists of 2 744 utterances with 19 511 word tokens from 1 660 word types.
3. Annotations
3.1. Orthographic transcription
For the RSC and CC, we used the original reading material to create a first transcription of the utterances which was subsequently corrected by the second author.
For the CSC, six linguistically trained transcribers created orthographic transcriptions manually. The transcribers participated in two specific training units of three hours each. In the first unit, they got familiar with the guidelines and they all started to annotate the same stretch of conversational speech. Two weeks later, in the second unit, they corrected the transcriptions of that stretch of speech under supervision of the first author and frequent mistakes were discussed together. Only then, they created the orthographic transcriptions of the other conversations. Finally, the transcriptions were corrected by a transcriber other than the one who created the first version. In this correction phase, they had to fill out a checklist for each file (e.g., Is the speaker audible on channel 1 transcribed in Tier 1? Are all overlapping words marked? Are all dialectal words tagged? etc.).
Transcribers used the open-source software PRAAT (Boersma, 2001), where for each speech file a TextGrid with separate tiers for each speaker was created. The details of the guidelines are strongly motivated by our experience on the automatic creation of phonetic and prosodic transcriptions, for which orthographic transcriptions are the basis (Schuppler et al., 2008; Gubian et al., 2009; Schuppler et al., 2011). Thus, the speech is segmented into short chunks (max 4 seconds, or longer only if no phrase boundary occurred) and the transcriptions contain a very detailed annotation of all speech and non-speech noises. The chosen set of symbols is similar as presented in the guidelines of the BAS project (Schiel et al., 2012). The complete set of symbols used for the orthographic transcription can be found in Appendix A.
With the orthographic transcriptions, we did not only aim at creating a good starting point for the automatic creation of further transcription layers, but we also aimed to create a good starting point for the analysis of conversational speech structures. The transcriptions contain a detailed annotation of backchannels (e.g., hm), of fillers (e.g., eh, ah, uh), repetitions and of broken words. Furthermore, we annotated overlapping speech: Already from the relative time intervals of the speech chunks one can calculate how long the overlapping intervals are, it is, however, not directly extractable which words overlap. We thus marked the overlapping words in the orthographic transcription (see Figure 2). Another focus was the transcription of laughter, where we distinguished laughter without linguistic content and words produced while laughing, or even while singing and laughing. Finally, we also annotated clearly audible breathings, and distinguished whether they were inbreaths or outbreaths. As far as punctuation is concerned, we decided not to set commas where the grammar would require it, but at the position of main phrase boundaries. Exclamation and question marks were used at the end of commands and questions, respectively.
The speech of all components and of all speakers is available with the manually created and corrected orthographic transcriptions.
3.2. Automatic phonetic transcription
Since the creation of phonetic transcriptions is very time and money consuming, we had to create the transcriptions automatically. We developed a two-step transcription procedure3 . In the first step, the tool uses a forced alignment with a pronunciation lexicon with multiple variants per word type. These variants are created by applying a set of 32 rules to the canonical pronunciation of the words. The rules cover co-articulation and reduction rules (e.g., schwa- deletion) which are typical for spontaneous German in general, and rules which are specific for Austrian German (e.g., /a/ realized as /o/ in stressed syllables, monophthongations and diphthongations). Furthermore, pronunciation variants have been created manually for the most frequent 150 words. Part of the automatically transcribed material (13091 segments,which is in range of the amount of data used to evaluate the PRAAT easy align tool, namely 9651 segments (Goldman, 2011)) was corrected manually and compared with the automatic transcription. Overall, there was a 18.5% discrepancy between the phone labels, which is in range with earlier studies on automatic transcriptions of spontaneous Dutch (24.3% in Cucchiarini and Binnenpoorte (2002)) and higher than the inter-transcriber agreement of spontaneous American English (between 73% and 76% in Raymond et al. (2002)). Finally, we used the manual corrections to improve our transcription procedure: we adapted our rules (e.g., reduce schwa deletion to certain contexts) and added variants to the lexicon which were far from the canonical form and could not be created by an application of rules.
In the second step, the tool annotates plosives on the sub-phonemic level: a burst detector determines whether a burst exists and where it is located. Plosives can thus be transcribed as either consisting of a closure and a burst, of only a closure or of only a burst. Again we validated the quality of the burst detector with manually corrected speech material. It outperforms previous tools with accuracies between 98% (for /t/s) and 74% (for /k/s) in read speech, and between 82% (for /g/s) and 52% (for /b/s) for conversational speech. After both steps, the automatic phonetic transcription as well as the sub-phonemic plosive annotation can be exported into a single PRAAT TextGrid. An example transcription of the CSC is shown in Figure 3. It shows the realization of the utterance eine Zeit lang ‘for some time’. Instead of the canonical form ["aI-n@ "tsaIt "laN], the speaker produces ["a "tsaIt "laN], where the pronunciation of the undefinite article eine is by far more frequent in conversational Austrian German than the canonical form (Schuppler et al., 2014a). At this point, the speech of all components of 12 speakers have been segmented automatically.
3.3. Prosodic annotation
Several prosodic annotation systems have been developed for German. These systems are often incompatible with one another, as they are based on different phonological models of German intonation (e.g., GToBI (Grice and Baumann, 2002), KIM (Kohler, 1991) and DIMA (K¨ugler et al., 2015)). Since the primary application for the prosodic annotation of the GRASS corpus is the creation of a prosody-dependent ASR system, our annotation system needs to fulfill the following requirements: (1) The manual annotation needs to serve as a basis to train an automatic annotation tool. (2) Thus, the annotation needs to be synchronous with the events in the speech signal. (3) Relationships between prosody and pronunciation variation and reduction must be easily extractable from the annotation. For these reasons, we decided to base our system on KIM, with some modifications/simplifications. The choice for KIM has another advantage: already large amounts of German spontaneous speech have already been annotated based on KIM and thus can be used as further training/testing material for our automatic annotation tool.
KIM is based on a separation of stress from intonation (Kohler, 1991). Lexical stress is labeled as primary or secondary and sentence stress is labeled from 0 to 3. The tonal peaks and valleys are annotated according to their position relative to the associated stressed vowel. Peaks are either early ‘)’, medial ‘ˆ’ or late ‘(’, while valleys are either early ‘]’ or late ‘[’. Phrase boundaries are labeled with various information: speech rate and reduction, scaling of the f0-endpoint, utterance final-lengthening and pause length.
Figure 4 shows an example of the prosodic annotation of an utterance taken from the CSC. The first tier contains the orthographic transcription and the second one the utterance split into its separate lexeme-units. Multiword expressions which are highly reduced are annotated as one lexeme-unit. In the example shown, the expression so wie ich den ‘as far as I’ is highly reduced and the speaker articulates only two syllables [so-"wIn]. German compounds, on the other hand, may be annotated as separate lexeme-units (see Figure 5), if non-initial stresses also carry accent (i.e., relevant pitch movement).
The third tier contains the annotation of the stress layer. The force of the stress is indicated by numbers from 0 to 3, where 0 corresponds to no stress at all and 2 to a standard accent. For reinforced stress, as used for contrastive focus, there is also stress level 3. Figure 6 shows an example utterance where stress level 3 occurs. The symbol after the number indicates the pitch movement (as in KIM). Whereas in KIM no pitch movement can be assigned to unstressed syllables, we allow that option in order to be able to mark early peaks. Figure 7 shows an example of the read speech component (RSC). Early peaks are typically used in read speech. We also found early peaks in the CSC, but only in cases where the read speech style was imitated. In Figure 7, the last and highest peak of the utterance is on the unstressed element das ‘the’ right before the pitch falls sharply to a valley on the clearly stressed first syllable of Wasser ‘water’. In such cases, we use the label ‘0ˆ’ to describe the temporal alignment without making assumptions about the segmental association of the tone (as e.g., GToBI would do with H+L* ). Table 7 in Appendix B shows all symbols used in this classification.
The fourth tier contains prosodic phrase boundaries within the utterance. In the annotation, we do not di↵erentiate between intonational and intermediate phrase boundaries, as it is required by GToBI. The example given in Figure 4 consists of two phrases. Although phrase boundaries sometimes coincide with syntactic boundaries, in our annotations they are defined strictly prosodically. Typical features indicating a phrase boundary are pause, final lengthening, change in speed rate, f0 contour, glottalization, etc. The annotation of the fourth tier contains three labels4 . The first digit indicates different types of speech rate and reduction (0-3), the second digit indicates the type of final-lengthening immediately preceding the boundary (0-2) and the third symbol indicates the type of f0 movement at the end of the phrase (‘,’, ‘.’, ‘?’). The example in Figure 4 shows a typical utterance. Whereas the first phrase shows a higher degree of reduction and ends with a high rise, the second phrase indicates the termination of the whole utterance with a final f0 fall. Both phrases are of medium overall speed and have default final lengthening. Table 8 in Appendix B shows all symbols used for this classification.
Finally, we annotated a fifth tier, which contains micro-prosodic features as listed in Table 9 in Appendix B. Figure 8 shows an example from the CSC which consists of two phrases. The first ends with a lengthening of the accented vowel in so ‘so’, annotated in tier 5 with the micro-prosodic label ‘L’. Since the lengthened vowel of this example is phrase-final, it is also annotated in the fourth tier (second digit = 2). The second phrase contains two highly reduced lexical-units (es ist so ein ‘it is such a’ pronounced as [@zi:-soa] and als was ’than the one’ as [@s-w@s]), and thus is labeled as having a ‘higher degree of reduction’ (fourth tier, first digit = 1). Since the vowels of the following two lexical-units du anhast ‘you are wearing’ are realized with creaky voice, they are labeled as ‘CV’ on the fifth tier.
At this point, 93 read sentences of three female and four male speakers and two hours of conversation from gender-mixed speaker pairs have been manually annotated prosodically. Manual annotations were made by the third author and all unclear cases were discussed with the first author. Subsequently, the third author corrected all manual annotations.
4. Characteristics of the Conversational Speech Component (CSC)
With the aim of recording natural, free, and casual conversations, we chose speaker pairs who knew each other very well (i.e., good friends, family members, couples). As mentioned earlier (cf. Section 2.3), only one quarter of the pairs started their conversation with the pictures provided, after a short warming up period, they spoke freely and casually. In this section, we focus on characteristics of the speech recorded which may give us an insight into the naturalness and casualness of the conversations. All numbers presented here were calculated on the basis of the annotations presented in the previous section.
4.1. Overlapping speech, disfluencies and laughter
4.1.1. General observations
In total, 55 571 chunks were recorded, of which actually 46 646 contain lexical items. Chunks without lexical items consist exclusively of sighs, breathing, smacking, etc. In total, 6 165 chunks contain one of these speaker noises. 508 chunks contained lexical items which were not intelligible for the transcriber. One of the most salient characteristics of free conversations is that people speak in overlap (e.g., Sacks et al. (1974); Schegloff (2000)). In our material, 43.5% of the chunks containing lexical items are (at least partly) spoken in overlap with the speech or laughter of the second speaker. The amount of overlap varies for the different conversations with a minimum of 18.7% and a maximum of 66.4% for the different conversations. This average percentage of overlap is slightly higher than what Schuppler et al. (2011) reported for the Ernestus Corpus of Spontaneous Dutch (38.1%) and what Chino and Tsuboi (1996) reported for a corpus of Chinese telephone dialogues (40%). Moreover, our speakers laughed frequently during the conversations: On average, 13.2% off all chunks contain pure laughter or lexical items produced while laughing, with a minimum of 1.5% and a maximum of 24.4% for the different conversations. The proportion of laughter observed in the GRASS corpus is on average in the range of what also Truong and Trouvain (2012) reported for conversational speech corpora.
Another indicator for the casualness of the conversations are disfluencies, which have been shown to be more frequent in casual conversations speech than in more formal speaking styles (e.g., Kohler et al. (2001); Shriberg (2001)). In our corpus, we observed a high number of broken words (1675), repeated lexical tokens (5 041) and hesitations such as eh, ah, ahm, ¨ah and ¨ahm (2 762). For the different conversations, the rate of broken words ranged between 0.16 and 1.7 broken words per 100 word tokens, the rate of repeated lexical tokens ranged between 0.8 and 4.9 and the rate of fillers ranged between 2.8 and 4.4. The overall filler rate in our corpus (3.5 fillers per 100 words) is higher than what Bortfeld et al. (2001) reported for a corpus of American English conversations (i.e., 2.6 of fillers eh, ah, uh per 100 words). The average total disfluency rate of 4.8 (broken words, repetitions and fillers) is in range of what Shriberg (2001) reports for spontaneous conversation (below 1.0 for human-computer dialog, up to 10.0 for natural conversations).
4.1.2. The role of the conversation partner
As mentioned above, the amount of overlap, laughter and disfluencies varies substantially between the different conversations. Table 2 shows an overview of the proportions of overlap, laughter and disfluencies separately for the different conversations grouped by their gender constellation (male male, male - female, female - female) and the type of relationship between the speakers (colleagues, friends, family members, couples).
In order to find out whether observed differences between the conversations are significant, we ran linear regression models using the R statistical package, following the procedure suggested by Levshina (2015). We built separate models for the different dependent variables: the amount of overlap, laughter, broken words, fillers and repetitions, as well as the total number of word tokens per 60 minutes of conversation (range between 5 106 and 15 063, mean = 11 238). The independent variables were gender and relationship, as well as their interaction-term. When building the models, we first added both variables and their interaction to the model and then removed not-significant predictors, but only if the removal decreased the AIC value (Akaike information criterion) of the model, and thus increased the quality of the model. In the following, we present the estimates, t-values and p-values for the significant predictors5 .
The model for overlap had two significant predictors: gender and relationship. In our data, conversations between female speakers (mean = 59.9%) contain significantly more overlapping speech than conversations between men (mean = 44.0%, ß= "0.17, t = -2.52, p < 0.05) and than gendermixed conversations (mean = 33.7%, ß = -0.20, t = -3.17, p < 0.01). Furthermore, conversations between colleagues (mean = 54.7%) contain significantly more overlapping speech than conversations between friends (mean = 41.4%, ß= -0.18, t = -2.32, p < 0.05) or family members (mean = 38.7%, ß= -0.27, t =-3.08, p < 0.01). There was no significant difference between pairs and colleagues regarding the amount of overlapping speech.
The model for broken words had one significant predictor: relationship. Conversations between family members contained significantly fewer broken words (mean = 0.38 broken words/100 word tokens) than conversations between colleagues (mean = 1.08, ß= -0.0065, t = -2.68, p < 0.05). The differences between pairs and friends were not significant. Furthermore, also the number of word tokens was significantly predicted by relationship: Conversations between family members (mean = 9439.7 word tokens per hour of conversation, ß= -4739.1, t = -2.45, p < 0.05) and pairs (mean = 8507.7, ß= -7082.5, t = -3.03, p < 0.01) contained significantly fewer word tokens than conversations between colleagues (mean = 13683.3). The differences between the conversations in terms of repetitions and fillers were not significant. Furthermore, in none of the models there were significant interactions between gender and relation.
For the model of laughter, we also tested whether the amount of repetitions, fillers, broken words and word tokens spoken condition the amount of laughter in a conversation. First, there is significantly less laughter in conversations with a higher number of words spoken in 1h of speech ( ß= -1.34e -05, t = -3.16, p < 0.001). This is somehow logical, given the more time speakers spend on laughing the less time there is left for producing lexical items. Second, we observed significantly more repetitions the higher the amount of laughter in a conversation ( ß= 2.97e + 00, t = 3.08, p < 0.001). Concerning the e↵ect of the conversation partner, the proportion of laughter is significantly higher in gender-mixed conversations (mean =11.01% , ß= 5.59e -02, t = 2.55, p < 0.01) than in conversations between women only (mean = 6.51%). There was no significant difference between conversations of men with men and women with women (mean = 6.47%).
In sum, conversations between female speakers contain significantly most overlapping speech and gender-mixed conversations contain significantly most laughter. As far as the relationship between the conversation partners is concerned, conversations between colleagues contain significantly most overlapping speech and the highest number of word tokens spoken by hour of conversation. Furthermore, we observed the significantly lowest proportion of broken words in conversations between family members.
4.2. Lexical items and non-standard lexicon
Since speakers chose their conversation topics freely, the Conversational Speech Compnent contains a broad lexicon covering many different topics, resulting in 198 129 word tokens from 13 231 different word types. 508 utterances partly contained lexical content which was not intelligible for the transcribers. This number is higher than previously reported for spontaneous conversations. For instance, the Ernestus Corpus of Spontaneous Dutch contains 115 chunks with unintelligible speech in 15h of recordings (Schuppler et al., 2011). Also representative for the conversational speech style is the high number of backchannels (hm, mhm, 4152 tokens) and backchannel like acknowledgment tokens (O.K, ja ‘yes’, nein ‘nein’, genau ‘exactly’, etc., in total 13 897 tokens), which together already make 9.11% of all tokens produced in the CSC (see Table 3 for details).
Also the use of colloquial vocabulary and dialectal words is representative for the casual, non-formal speech register (Torreira et al., 2010). We call those words colloquial which are typical for non-formal conversations, but would also occur in other German varieties. We call those words dialectal which do not have a normed orthographic form in Standard Austrian German6 . In total, the transcribers annotated 1203 word tokens of 378 different word types as dialectal. The 12 most frequent dialectal words (shown in Table 4) already make 48.3% of all dialectal word tokens.
One of the most frequent words in the corpus is halt (1358 tokens), a very common modal particle for casual conversations in the southern German varieties. It mostly corresponds to the Standard German words nun einmal or eben (e.g., as in Es ist halt so ‘That’s just the way it is’), which are, as can be expected given the regional background of the speakers, much less frequent in our corpus (nun einmal (3 tokens), eben(347 tokens)). Similarly, the word kriegen ‘to receive’ and its verbal forms (211 tokens) is much more frequent than all of its Standard German synonyms together (bekommen ‘to receive’ (7 tokens), erhalten ‘receive’ (1 token), erreichen ‘reach’ (4 tokens), mitbekommen ‘to understand’ (1 token); other synonyms as for instance versehen, zuziehen, verschaffen ‘to occupy, to contract an illness, to procure something’ do not occur at all). The high frequency of halt and kriegen are clear indicators for the casual speaking style.
A preposition which is exclusively used in casual Viennese to emphasize adjectives is ur- (15 tokens), of similar low frequency are the dialectal emphasizers gescheit ‘absolutely’7 (12 tokens) and the rather vulgar emphasizer sau- (15 tokens). These emphasizers may be used with positive adjectives as in urgem¨utlich ‘really cosy’ or with negative ones as in saukalt ‘extremely cold’. The way more frequent strategy for emphasizing adjectives, however, is the use of the adverbs echt ‘really’ (480 tokens), ganz ‘totally’ (389 tokens), voll ‘really’ (323 tokens), its variant volle (12 tokens), richtig ‘really’ (117 tokens) and total ‘totally’ (105 tokens). These adverbs are typical for informal spoken German in general, not only in Austrian German.
Finally, also the use of swearwords is typical for casual conversations. Eggins and Slade (1997) mention that the use of swearwords is an evidence for a casual speaking style. This is also the conclusion drawn by Torreira et al.(2010), who compared the use of swearwords in two corpora of spontaneous French, the Nijmegen Corpus of Casual French (NCCFr) and the ESTER corpus of journalistic speech. They find that swearwords are highly frequent in the casual material of the NCCFr, where for instance the word putain occurs once every six minutes. In comparison to the frequency of swearwords in the NCCFr, the number of swearwords is much lower in the GRASS corpus. We counted a total of only 154 swearwords from 46 different types. We found swearwords of the type as also typical in conversations of other German varieties (e.g., Arschloch ‘asshole’ (1 token), Scheiße ‘shit’ and its compounds (69 tokens)), and dialectal ones (e.g., deppert ‘stupid’ (27 tokens), Schaß ‘shit’ (7 tokens) and Trottl ‘idiot’ (7 tokens)). One hypothesis why swearwords occur less frequently in our data is that our speakers are much older (age on average: 33.8 years) than the students recorded in NCCFr (average: 22.2 years). This hypothesis is also supported by the literature on swearing in natural conversations, which in general shows that the informal speech vocabulary of college students is disproportionally high in the occurrence of profanity (Beers F¨agersten, 2012). In sum, the use of colloquial vocabulary, dialectal words and swearwords clearly indicate that the recording situation did not provoke the speakers to try to produce a formal speech register.
5. Conclusion
In this paper, we presented GRASS (Graz Corpus of Read and Spontaneous Speech), the first large scale speech database for Austrian German. It contains in total 30 hours of speech from 38 speakers from five different provinces of Austria, where for each speaker approximately 30 min of read speech and commands and 1h of free conversations were recorded. It has been transcribed manually at the orthographic level, with much detail on conversational aspects. Furthermore, new methods have been developed for its phonetic transcription at the segmental (i.e., broad phonetic transcription) and sub-segmental level (i.e., sub-segmental annotation of plosives). Finally, part of the read and conversational speech of the corpus was manually an-notated at the prosodic level. Here, we presented the prosodic annotation system, a simplified/modified version of KIM, and illustrated specific issues relevant for the transcription of conversational German. In the future, we plan to manually annotate the read and conversational speech of more speakers and to use these annotations to develop an automatic prosodic annotation tool for read and spontaneous Austrian German.
On the basis of our analysis of measures such as filler-rates, disfluencyrates, the amount of laughter and overlapping speech in the conversations and the amount of colloquial and dialectal words, we came to the conclusion that the recorded conversations are truly casual. We believe that this casualness was achieved because the conversations were between speakers who knew each other very well (family members, couples, friends, colleagues), because no experimenter was present during the recordings and because the speakers did not receive instructions to talk about a specific topic. The material is in this respect not only a valuable resource for speech scientists studying Austrian German, but also for those interested in natural conversations in general. Moreover, the speech is rich in pronunciation variation, given that we recorded speech from different speaking styles and of speakers from different regional backgrounds. The corpus may thus be the basis for future phonetic and socio-phonetic studies as well as for the development of ASR and dialogue systems for Austrian German.
Corpus Availability
The corpus-webpage, which can be found at www.spsc.tugraz.at, provides more details about the collection of the GRASS corpus along with audio and transcription examples as well as information about ongoing work. This webpage also informs about how to obtain a copy of the corpus, meta-data on the speakers, the pronunciation lexicon and tools for searching the corpus. In general, the corpus is available for all Universities and non-commercial research institutes.
Acknowledgements
The work by Barbara Schuppler and by Alexander Zahrer was supported by the Austrian Science Fund (T572N23). The manual creation of the prosodic annotations were partly funded by and Initial Funding Grant from the Graz University of Technology (AF3-442-01). The work of Martin Hagm¨uller was partly funded by the European project DIRHA (FP7-ICT-2011-7-288121). We would like to thank Margaret Zellers for her advice with the prosodic annotation system and for her comments on one of the earlier versions of this manuscript.
References
Adda-Decker, M., Schuppler, B., Lamel, L., Morales-Cordovilla, J. A., Adda, G., 2013. What we can learn from ASR errors about low-resourced languages: a case-study of Luxembourgish and Austrian. In: Errare Workshop 2013. LIMSI, Paris, France.
Auer, P., Hinskens, F., Kerswill, P., 2008. Dialect Change. Convergence and Divergence in European Languages. Cambridge University Press, Cambridge, UK.
Baum, M., Erbach, G., Kubin, G., 2000. SpeechDat-AT: A telephone speech database for Austrian German. In: Proceedings of the LREC Workshop: Very Large Telephone Databases (XLDB). pp. 51–56.
Beers F¨agersten, K., 2012. Who’s Swearing Now? The Social Aspects of Conversational Swearing. Cambridge Scholars Publishing, Newcastle upon Tyne, UK.
Boersma, P., 2001. Praat, a system for doing phonetics by computer. Glot International 5, 314–345.
URL http://www.fon.hum.uva.nl/praat/ (last viewed 18-01-2017)
Bortfeld, H., Leon, S. D., Bloom, J. E., Schober, M. F., Brennan, S. E., 2001. Disfluency rates in conversation: E↵ects of age, relationship, topic, role and gender. Language and Speech 44 (2), 123–147.
Burger, S., Schiel, F., 1998. RVG 1 - A database for regional variants of contemporary German. In: Proceedings of LREC. pp. 1083–1087.
Caren Brinckmann, Stefan Kleiner, R. K., Berend, N., 2008. German Today:a really extensive corpus of spoken standard German. In: Proceedings of LREC. pp. 3185 – 3191.
Chino, T., Tsuboi, H., 1996. A new discourse structure model for spontaneous spoken dialogue. In: Proceedings of ICSLP. pp. 1021–1024.
Cucchiarini, C., Binnenpoorte, D., 2002. Validation and improvement of automatic phonetic transcriptions. In: Proceedings of ISCLP. Denver, USA, pp. 313–316.
D¨urrscheid, C., Elspaß, S., 2015. Variantengrammatik des Standarddeutschen. In: Kehrein, R., Lameli, A., Rabanus, S. (Eds.), Regionale Variation des Deutschen. Projekte und Perspektiven. de Gruyter, Berlin/Boston.
Eggins, S., Slade, D., 1997. Analysing Casual Conversation. Equinox Publishing Ltd., London, UK.
Ernestus, M., 2000. Voice assimilation and segment reduction in casual Dutch. A corpus-based study of the phonology-phonetics interface. Ph.D. thesis, LOT, Vrije Universiteit Amsterdam, The Netherlands.
Feiser, H., 2015. Untersuchung auditiver und akustischer Merkmale zur Evaluation der Stimm¨ahnlichkeit von Br¨uderpaaren unter forensischen Aspekten. Ph.D. thesis, Institut f¨ur Phonetik und Sprachverarbeitung, LudwigMaximilians-Universit¨at M¨unchen.
Goldman, J.-P., 2011. EasyAlign: a friendly automatic phonetic alignment tool under Praat. In: Proceedings of Interspeech. pp. 3233 – 3236.
Grice, M., Baumann, S., 2002. Deutsche Intonation und GToBI. Linguistische Berichte 191, 267–298.
Gubian, M., Schuppler, B., van Doremalen, J., Sanders, E., Boves, L., 2009.Novelty detection as a tool for automatic detection of orthographic transcription errors. In: Proceedings of SPECOM. pp. 509–514.
H¨ansler, E., Schmidt, G., 2004. Acoustic Echo and Noise Control: A Practical Approach. Wiley- IEEE Press.
Hobel, B., Vollmann, R., 2015. Phonological case study of the use of (Styrian) dialect and standard language in German as second language. Grazer Linguistische Studien 84, 5–20.
IPDS, 1997. CD-ROM: The Kiel Corpus of Spontaneous Speech, vol i- vol iii. Corpus description available at http://www.ipds.unikiel.de/forschung/kielcorpus.de.html (last viewed 03/11/2016).
Klaaß, D., 2008. Untersuchungen zu ausgew¨ahlten Aspekten des Konsonantismus bei ¨osterreichischen Nachrichtensprechern. Duisburger Papers on Research in Language and Culture 74, 7–277.
Kohler, K. J., 1991. A model of German intonation. In: Kohler, K. J. (Ed.), Studies in German Intonation, AIPUK 25. IPDS, pp. 51–67.
Kohler, K. J., Peters, B., Wesener, T., 2001. Phonetic exponents of disfluency in German spontaneous speech. In: Diss01 - Disfluency in Spontaneous Speech. pp. 45–48.
K¨ugler, F., Smolibocki, B., Arnold, D., Baumann, S., Braun, B., Grice, M., Jannedy, S., Michaelsky, J., Niebuhr, O., Peters, J., Ritter, S., R¨ohr, C. T., Schweitzer, A., Schweitzer, K., Wagner, P., 2015. DIMA annotation guidelines for German intonation. In: Proceedings of ICPHS. p. 317.
Levshina, N., 2015. How to do linguistics with R. Data exploration and statistical analysis. John Benjamins Publishing Company, Amsterdam/Philadelphia.
Leykum, H., Moosm¨uller, S., Dressler, W. U., 2015. Homophonous phonotactit and morphonotactic consonant clusters in word-final position. In: Proceedings of Interspeech. pp. 1685–1689.
Moosm¨uler, S., Ringen, C., 2004. Voice and aspiration in Austrian German plosives. Folia Linguistica 38, 43–62.
Moosm¨uller, S., 1997. Diphthongs and the process of monophthongization in Austrian German: A first approach. In: Proceedings of Eurospeech. pp. 787–790.
Moosm¨uller, S., 1998. The process of monophthongization in Austria (reading material and spontaneous speech). In: Papers and Studies in Contrastive Linguistics. pp. 9–25.
Moosm¨uller, S., 2007. Vowels in Standard Austrian German. An AcousticPhonetic and Phonological Analysis. Habilitation, University of Vienna.
Moosm¨uller, S., Dressler, W. U., 1988. Hochlautung und soziophonologische Variation in ¨Osterreich. Jahrbuch f¨ur Internationale Germanistik 20 (2), 82–90.
Muhr, R., 2000. ¨Osterreichisches Sprachdiplom Deutsch. Lernzielkataloge.¨obv und hpt, Wien.
Muhr, R., 2007. ¨Osterreichisches Aussprachew¨orterbuch – ¨Osterreichische Aussprachedatenbank. Peter Lang Verlag, Frankfurt/M., Wien u.a. 525 S. mit DVD.
Muhr, R., 2008. The pronouncing dictionary of Austrian German (AGPD) and the Austrian phonetic database (ADABA): Report on a large phonetic resources database of the three major varieties of German. In: Proceedings of LREC. pp. 3093–3100.
Pitt, M. A., Johnson, K., Hume, E., Kiesling, S., Raymond, W. D., 2005.The Buckeye corpus of conversational speech: Labeling conventions and a test of transcriber reliability. Speech Communication 45, 89–95.
Raymond, W. D., Pitt, M., Johnson, K., Hume, E., Makashay, M., Dautricourt, R., Hilts, C., 2002. An analysis of transcription consistency in spontaneous speech from the Buckey Corpus. In: Proceedings of ICSLP. pp. 2466–2469.
Sacks, H., Scheglo↵, E. A., Je↵erson, G., 1974. A simplest systematics for the organization of turn-taking for conversation. Language 50, 696–735.
Sauer, S., Rasskazova, O., February 2014. Bematac: eine digitale multiomodale Ressource f¨ur Sprach- und Dialogforschung. In: Workshop: Grenzen berschreiten - Digitale Geisteswissenschaft heute und morgen, Digital Humanities. Berlin, Germany.
Schegloff, E. A., 2000. Overlapping talk and the organization of turn-taking for conversation. Language in Society 29, 1–63.
Schiel, F., Draxler, C., Baumann, A., Ellbogen, T., Ste↵en, A., 2012. The production of speech corpora, version 2.5. Tech. rep., Bavarian Archive for Speech Signals, University of Munich.
Schiel, F., Heinrich, C., Barf¨ußer, S., Gilg, T., 2008. ALC: Alcohol Language Corpus. In: Proceedings of LREC. pp. 1465–1470.
Schuppler, B., 2011. Automatic analysis of acoustic reduction in spontaneous speech. Ph.D. thesis, Radboud University Nijmegen, The Netherlands.
Schuppler, B., Adda-Decker, M., Morales-Cordovilla, J. A., 2014a. Pronunciation variation in read and conversational Austrian German. In: Proceedings of Interspeech. pp. 1453–1457.
Schuppler, B., Ernestus, M., Scharenborg, O., Boves, L., 2008. Preparing a corpus of Dutch spontaneous dialogues for automatic phonetic analysis. In: Proceedings of Interspeech. pp. 1638–1641.
Schuppler, B., Ernestus, M., Scharenborg, O., Boves, L., 2011. Acoustic reduction in conversational Dutch: A quantitative analysis based on automatically generated segmental transcriptions. Journal of Phonetics 39, 96–109.
Schuppler, B., Grill, S., Menrath, A., Morales-Cordovilla, J. A., 2014b. Automatic phonetic transcription in two steps: forced alignment and burst detection. In: Besacier, L., Dediu, A., Mart´ın-Vide, C. (Eds.), Statistical Language and Speech Processing. SLSP 2014. Lecture Notes in Artificial Intelligence. Vol. 8791. pp. 132–143.
Schuppler, B., Hagm¨uller, M., Morales-Cordovilla, J. A., Pessentheiner, H., 2014c. GRASS: the Graz corpus of Read And Spontaneous Speech. In: Proceedings of LREC. pp. 1465–1470.
Shriberg, E., 2001. To ‘errrr’ is human: ecology and acoustics of speech disfluencies. Journal of the International Phonetic Association 31 (1), 153169.
Steiner, E., Vollmann, R., 2010. Fragebuch zur Sprachdatenerhebung in der Steiermark. Tech. rep., Karl-Franzens Univ. Graz.
Torreira, F., Adda-Decker, M., Ernestus, M., 2010. The Nijmegen Corpus of Casual French. Speech Communication 52 (3), 201–212.
Truong, K. P., Trouvain, J., 2012. On the acoustics of overlapping laughter in conversational speech. In: Proceedings of Interspeech. pp. 459–462. Vollmann, R., Moosm¨uller, S., 2001. ’Nat¨urliches Driften’ im Lautwandel: die Monphthongierung im ¨osterreichischen Deutsch. Zeitschrift f¨ur Sprachwissenschaft 20 (1), 42–65.
Weilhammer, K., Reichel, U., Schiel, F., 2002. Multi-tier annotations in the Verbmobil Corpus. In: Proceedings of LREC. pp. 912–917.
William, L., 2001. Principles of Linguistic Change: Vol.2. Social Factors.Wiley-Blackwell.
APPENDIX A
For the creation of the orthographic transcriptions, transcribers used the set of symbols shown in Table 5. While creating the transcriptions, the transcribers compiled a lexicon with the words which do not already have an entry in the ADABA lexicon (Lexicon of standard Austrian German (Muhr, 2007)). This lexicon is shared among the transcribers (see column ‘Lexicon’ in Table 5).
APPENDIX B
The following tables show all symbols used for the prosodic transcription, separately for the different tiers.
1Non-standard abbreviations used in the paper: CC: Commands Component, CH: chair, CSC: Conversational Speech Component, DAW: digital audio workstation, FM: large diaphragm fixed microphone, GRASS: Graz Corpus of Read and Spontaneous Speech, HM: head mounted microphone, LG: laryngograph, MS: music stand, RSC: Read Speech Component, SC: screen, T: table, V: video.
2The recording procedure and the orthographic transcription of GRASS have earlier been presented at LREC (Schuppler et al., 2014c). Here, we provide more details on the creation of the orthographic transcription, the phonetic and prosodic annotation layers, as well as an analysis of the casualness of the Conversational Speech Component.
3A full description of an earlier version of the transcription tool has been presented at the SLSP conference (Schuppler et al., 2014b). The transcription system, however, was not yet improved on the basis of the manually corrected automatic phonetic transcriptions.
4These are the same as the first, second and fifth symbol of the KIM annotation system.
5We assume the following significance levels, which are marked with stars in Table 2: highly significant (***): p < .001; significant (**): p < .01; significant (*): p < .05; and marginally significant (.): p < .1.
6For instance, the Austrian word Karfiol for the German Standard Blumenkohl ‘cauliflower’ is not considered dialectal.
7In the standard language it is and adjective meaning ‘clever’.
8Not in the sense of mein ‘mine’.
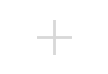