Arxiv NLP 0114 Papers
356 阅读 2020-07-14 14:54:02 上传
以下文章来源于 语言学之妙
Alibaba 1 篇:[3]
Carnegie Mellon University 1 篇:[8]
Google 3 篇:[10], [13], [21]
Berkeley 1 篇:[13]
https://arxiv.org/abs/2001.04362
Han Guo, Ramakanth Pasunuru, Mohit Bansal
AAAI 2020 (9 pages)
https://arxiv.org/abs/2001.04351
Liang Xu, Qianqian Dong, Cong Yu, Yin Tian, Weitang Liu, Lu Li, Xuanwei Zhang
CLUE Organization
6 pages, 5 tables, 1 figure
https://arxiv.org/abs/2001.04246
Daoyuan Chen, Yaliang Li, Minghui Qiu, Zhen Wang, Bofang Li, Bolin Ding, Hongbo Deng, Jun Huang, Wei Lin, Jingren Zhou
Alibaba Group
https://arxiv.org/abs/2001.04200
Tianjun Hou, Bernard Yannou, Yann Leroy, Emilie Poirson
https://arxiv.org/abs/2001.04170
Yohan Chalier, Simon Razniewski, Gerhard Weikum
T´el´ecom ParisTech
11 pages
https://arxiv.org/abs/2001.04063
Yu Yan, Weizhen Qi, Yeyun Gong, Dayiheng Liu, Nan Duan, Jiusheng Chen, Ruofei Zhang, Ming Zhou
https://arxiv.org/abs/2001.03897
Elham Seifossadat, Hossein Sameti
Sharif University of Technology; our model on nine domains from tabular, dialogue act and RDF format. Our model outperforms; the corpus-based state-of-the-art methods trained on tabular datasets and also achieves compa-; rable results with neural network-based approaches trained on dialogue act, EE and WebNLG; datasets for BLEU and ERR evaluation metrics. Also, by reporting Human Evaluation results,; we show that our model produces high-quality utterances in aspects of informativeness and; naturalness as well as quality.
https://arxiv.org/abs/2001.03844
Jinlan Fu, Pengfei Liu, Qi Zhang, Xuanjing Huang
(cid:) Language Technologies Institute, Carnegie Mellon University,
Accepted by AAAI 2020
https://arxiv.org/abs/2001.03830
Hongmin Wang
University of California Santa Barbara
Best Paper Runner-up at INLG 2019 (12th International Conference on Natural Language Generation)
https://arxiv.org/abs/2001.03765
Jeffrey Ling, Nicholas FitzGerald, Zifei Shan, Livio Baldini Soares, Thibault Févry, David Weiss, Tom Kwiatkowski
Google Research; † Work done as a Google AI Resident.
https://arxiv.org/abs/2001.03708
Jieh-Sheng Lee, Jieh Hsiang
PatentTransformer-; Department of Computer Science and Information Engineering; National Taiwan University, Taiwan
demo paper
https://arxiv.org/abs/2001.03632
R. Thomas McCoy, Robert Frank, Tal Linzen
Johns Hopkins University
12 pages, 10 figures; accepted to TACL
https://arxiv.org/abs/2001.04451
Nikita Kitaev, Łukasz Kaiser, Anselm Levskaya
U.C. Berkeley & Google Research
ICLR 2020
https://arxiv.org/abs/2001.04437
Vlad Niculae, André F. T. Martins
Instituto de Telecomunicações
43 pages, 5 tables, 4 figures
https://arxiv.org/abs/2001.04425
Hiba Arnaout, Simon Razniewski, Gerhard Weikum
Max Planck Institute for Informatics
10 pages
https://arxiv.org/abs/2001.04346
Xin Dong, Jingchao Ni, Wei Cheng, Zhengzhang Chen, Bo Zong, Dongjin Song, Yanchi Liu, Haifeng Chen, Gerard de Melo
Rutgers University, NEC Laboratories America
https://arxiv.org/abs/2001.04345
Mukul Kumar, Youna Hu, Will Headden, Rahul Goutam, Heran Lin, Bing Yin
Understanding⋆
https://arxiv.org/abs/2001.04260
Seung Hee Yang, Minhwa Chung
Interdisciplinary Program in Cognitive Science, Seoul National University; Department of Linguistics, Seoul National University, Republic of Korea
https://arxiv.org/abs/2001.04219
Markus Hecher, Michael Morak, Stefan Woltran
TU Wien, Vienna, Austria,
https://arxiv.org/abs/2001.04192
Rinaldo Lima, Bernard Espinasse, Fred Freitas
Departamento de Computação, Universidade Federal Rural de Pernambuco, Recife, Brazil; Aix-Marseille Université, LIS-UMR CNRS, Marseille, France; Centro de Informática, Universidade Federal de Pernambuco, Recife, Brazil
https://arxiv.org/abs/2001.03671
Harsh Mehta, Yoav Artzi, Jason Baldridge, Eugene Ie, Piotr Mirowski
Google Research
相关工具
点赞
收藏
表情
图片
附件
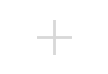