1382 阅读 2020-08-24 09:30:04 上传
以下文章来源于 十分语言学
编者按
《理论语言学五道口站》(2020年第37期,总第101期)理论与方法专栏为大家推荐美国伦斯勒理工学院认知科学系Marjorie McShan副教授和美国伦斯勒理工学院认知科学系系主任Sergei Nirenburg教授的著作:Linguistics for the Age of AI(《人工智能时代的语言学》)。本书将于2021年在MIT Press出版,欢迎各位学者关注。
著作简介
Linguistics for the Age of AI
(《人工智能时代的语言学》)
“人工智能”领域的初始研究目标之一就是使“智能代理系统”可以像人一样处理“自然语言”。近年来,该领域的研究重点主要放在“大数据统计分析”和“机器学习算法研发” 两个方面,而人类 “学习行为建模” 等方面很少涉及。本书作者Marjorie McShane和Sergei Nirenburg就“学习行为建模”这一方面进行了深入探究,希望在“智能代理系统”现有成果的基础上制造出一台真正拥有“人类水平”的智能机器。受人脑“信息处理系统”启发,他们为“智能代理系统”设计出一套“语言理解加工”的精密模型,希望该模型无论在书面语表达还是日常口语对话中,都能够依据具体语境、辨别出语言的“深层含义”。
书中,McShane和Nirenburg主要从“认知建模”和“系统构建”两个方面对“智能代理系统”的“语言分析能力”的构建进行了详细描述,其构建过程包括 “外在信息解读”、 “客观现象阐述” 和“深化理解学习”三个方面。他们还重点强调了“语言分析能力”构建的“可操作性”,要求其应该达到:内容“理解全面”、表达“言简意赅”和操作“合理有序”。此外,McShane和Nirenburg还在书中阐述了一些微观理论,如“语义分析”、“基础算法”和“情景推理”若干理论,该微观理论不仅对“语言分析能力”的构建具有重要参考价值,同时也是该能力构建的有效评价标准。
McShane和Nirenberg指出,如果想要开发出“与人类水平相当”的人工智能,唯一的办法就是把“语言学理论”放在首位,然后对大数据展开统计分析。McShane和Nirenberg在综合认知框架下制定了一个长期的研究计划,力求“语言学理论知识”和“客观数据分析”双管齐下。
One of the original goals of artificial intelligence research was to endow intelligent agents with human-level natural language capabilities. Recent AI research, however, has focused on applying statistical and machine learning approaches to big data rather than attempting to model what people do and how they do it. In this book, Marjorie McShane and Sergei Nirenburg return to the original goal of recreating human-level intelligence in a machine. They present a human-inspired, linguistically sophisticated model of language understanding for intelligent agent systems that emphasizes meaning—the deep, context-sensitive meaning that a person derives from spoken or written language.
With Linguistics for the Age of AI, McShane and Nirenburg offer a roadmap for creating language-endowed intelligent agents (LEIAs) that can understand, explain, and learn. They describe the language-understanding capabilities of LEIAs from the perspectives of cognitive modeling and system building, emphasizing “actionability”—which involves achieving interpretations that are sufficiently deep, precise, and confident to support reasoning about action. After detailing their microtheories for topics such as semantic analysis, basic coreference, and situational reasoning, McShane and Nirenburg turn to agent applications developed using those microtheories and evaluations of a LEIA's language understanding capabilities.
McShane and Nirenberg argue that the only way to achieve human-level language understanding by machines is to place linguistics front and center, using statistics and big data as contributing resources. They lay out a long-term research program that addresses linguistics and real-world reasoning together, within a comprehensive cognitive architecture.
注释
1. 智能代理(intelligent agent)是定期地收集信息或执行服务的程序,它不需要人工干预,具有高度智能性和自主学习性,可以根据用户定义的准则,主动地通过智能化代理服务器为用户搜集最感兴趣的信息,然后利用代理通信协议把加工过的信息按时推送给用户,并能推测出用户的意图,自主制订、调整和执行工作计划。
2. 机器学习(machine learning)是人工智能的一个研究分支,主要是设计和分析一些让计算机自动获取知识的算法,涉及到概率论、统计学等多个领域。
作者简介
- Marjorie McShane -

Marjorie McShane是美国伦斯勒理工学院认知科学系副教授、认知科学家和计算机语言学家。她开发出了智能代理系统的认知模型,该系统可以按照任务指令与人类进行对话。她的研究领域主要包括:语言学、认知建模、自然语言处理等。
- Niels O. Schiller -

Sergei Nirenburg是美国伦斯勒理工学院认知科学系教授兼系主任。他在认知科学、人工智能和自然语言处理领域有着30多年的工作经验,领导着80多人的研发团队。他的研究兴趣主要包括开发人类认知能力的计算模型,以及如何在现实社会中通过人工智能来实现该模型;“本体语义学”理论、知识的获取和管理等。
Sergei Nirenburg,professor & department head of the Cognitive Science Department at Rensselaer Polytechnic Institute. Sergei Nirenburg has worked in the areas of cognitive science, artificial intelligence and natural language processing (NLP) for over 30 years, leading R&D teams of up to 80. His professional interests include developing computational models of human cognitive capabilities and implementing them in hybrid-engine models of societies of human and computer agents; continued development of the theory of ontological semantics; and acquisition and management of knowledge about the world and about language.
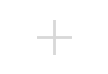