805 阅读 2021-04-25 09:48:11 上传
以下文章来源于 语言学考研
前言
首先,做nlp不一定要很懂语言学,也不一定要跟语言学扯上关系。nlp可以仅是data mining,features engineering, 也的确有很多work目前在用文本或者对话做为数据集,然后用统计学方法实现目的,比如deep learning 。在某些任务上统计学模型功不可没,比如machine translation, speech recognition, question answering, etc.
大多数人对nlp和语言学联系的了解,在于认为rule-based的nlp就是基于语言学。的确rule-based是语言学里广泛使用的,尤其是语法(syntax, syntactic structure)。现在machine learning的发展已经可以将rules转换为hidden states,人不用去操心提出大量rules来做exhaustive search。
但computational linguistics所包含的,远远大于rules。人类语言是漫长历史进化的高级产物,远不是成千上万个rules能描述清楚的。能被nlp利用的语言学,除了枚举rules外还有很多很多。
接下来的回答是给真正对computational linguistics和nlp本身感兴趣的,对某些语言现象感兴趣,并打算在这条路上开始钻研的同学的一些建议。(想忽略细节的同学请直接拉到答案最后找reference)
人大脑工作不是靠probablistic language modeling,咱们谁的脑袋里都不会听到一个词然后跑一遍hidden markov,毕竟也进化了这么多年了不是。
与nlp相关,跟概率论并进的,除了传统的语言学,还有logic呢,Lofti Zadeh老爷爷研究了一辈子的fuzzy logic,也是在探究semantics&world knowledge (再次感谢老爷爷的贡献,r.i.p)。
另外,语言学自身是个很大又很宽泛,又互相交叉的学科。有很多研究是跟literatures and arts有关,有的是跟cognitive science有关,还有neuroscience, mathematics, education, psychology, etc。我涉猎有限,在此只能回答跟computational linguistics有关("to the best of my knowledge")。
No.1
Definition(语言学对语言任务的定义)
在研究任何问题前,都必须要想清楚你的问题是什么,怎么定义。许许多多nlp research都是基于语言学上的定义,像我下文会提到的semantics, grammar。可是如果没有从沿用语言学的定义到nlp,这个0到1的过程,最早做researchers的人该如何想明白他们的research question?
做对话系统的同学应该很熟悉dialogue acts. 现在的对话系统发展迅猛,很多新应用都基于reinforcement learning, 并且取得显著成就。尤其是某些task-oriented dialogue generator, 早就不是十多年前的rule-based system了。但任何一个系统在设计之初都要采用dialouge acts定义(当然还有其他定义),来明确该系统的目的。不然该系统如何区分wh-question, yes-no question, greetings, 还有其他?(如果觉得见到“wh-”开头,问号结尾,就是一个wh-question rule, 那我不知道该说什么好了)
明确自己的research task并且贯彻到底是好事,如果要做language modeling,基于machine learning/deep learning, 那真的不用费时间在语言学上。但觉得语言学是rule based已经过时了被淘汰了,这个锅语言学真的背的有点冤呀。

阿里机器翻译能力示意图
No.2
Grammar(语法学)
Grammar是我会首先推荐的方向。Grammar分为morphology&syntax. 在这里我主要指syntax.细节可以看Chomsky, Michael Colins, Jason Eisner等人的工作。现在大家用的最多的应该是stanford的syntactic parsing吧。这方面的工作已经很成熟,要处理语言基本是拿来就能用了。但是语法树到底是什么,怎么构建,syntatic parsing优势,如何处理ambiguity, 想要做computational linguistics的话,这些很有必要知道。最基本的例子是,当用parser来处理你的句子,你起码要能看懂这个parser output是否make sense。

转换生成语法理论创始人乔姆斯基
No.3
Semantics(语义学)
这个部分是我做最多的,感觉也是被误解最多的。尤其推荐 “Meaning in language: An introduction to Semantics and Pragmatics.” 我并没有看完。Semantics是个很复杂的研究,可以涉及到语法,句法,world knowledge, 但最终还是回归semantics自身。目前nlp里很火的有distributional semantic representation (word embedding, phrase embedding, sentence embedding, etc), semantic parsing (logical form, etc), 等等等等。同一句话可以表达的意思太多了,同一个意思带来的表达形式也太多了。一个简单句子里包含的意思会涉及到当下对话双方的情景,以前或者以后会发生的事,等等。举个个人很喜欢的例子:

第一层意思是直观semantics, 能够被目前的semantic representation捕捉到。第二层是presupposition, 代表着在说话当下当事人双方默认已经发生的事情,是semantics研究中的难点;第三层包含了sentiment, 做情感分析的同学应该很了解,能否被目前的classifier捕捉到我不清楚。第四层是现在也很火的coreference resolution, 虽然原文里没有明确指代每个人称代词,但听众和当事人很直接能把每个人物代入,甚至包括Trump省略的"I will release (my taxes)". 目前的co-reference resolution,e.g. stanford corenlp, 可以解决前三个代词,但省略的部分似乎还做不到。
对Semantic要求最高也是最难的,在nlp中应该是在natural language understanding相关应用了。Semantics里包含了太多太多的现象,如果能稍微研究并且model其中一小部分,对downstream application来说都会是一个很大的boost。前段时间有个shared task,叫 "hedge detection",目的是找出文本信息中的hedges and cues。大部分人会关注这个shared task下哪个模型做的最好,个人认为难点是在定义。有“but”,"however"出现语意就一定转折了么?如果被转折,是所在句子,还是段落还是一个小phrase呢?有dependency存在么?另一个相似shared task是negation detection. 想要理解这些问题本身和其难点所在,computational linguistics的前期知识储备是并不可少的。
No.4
Pragmatics(语用学)
这里很重要的理论,是computational pragmatics范畴里的:Grice's maxims, 和Rational Speech Act(RSA). 这两个理论其实紧密相关。前者理论关于谈话双方为了有效沟通会有意识的遵守的一些principle, (同时可见“cooperative principle”), 后者关于为了达到这种有效沟通,对话当中存在的一种recursive process, 并且是bayesian inference. 如果你的工作跟 inference, reasoning相关,请一定要阅读。做对话系统的应该已经很熟悉了。
No.5
Fuzzy logic(模糊逻辑)
目前还是有researcher继承Zadeh老爷爷的衣钵,并且用fuzzy logic做出了很多natural language generation, information extraction方面的成就的。个人经验而言,我博士第一年(2014)一直在关注deep learning/machine learning方面,当时觉得它们是万能的。直到第二年夏天在忙一个project, 阅读了Zadeh老爷爷的大量工作,才感觉自己一直在以很片面的眼光看research。当时真的做了满满一本笔记。
后记
最后,如果兴趣在建modeling,deep learning architecture, 语言学方面的part-of-speech也好,parsing也好,都只是你的工具;
同样,如果兴趣在computational linguistics,语言现象,deep learning/machine learning都是你的工具。
取决与你的任务是什么,取决于你有没有完全dedicated的信心。毕竟巴菲特和Geff Hinton是少数,大多数人都无法预测20年后火的适合什么。
感谢阅读。希望能给在犹豫是否开始computational linguistics和nlp研究同学们一些帮助。
(任何不准确的地方还请大家指正)
推荐
Reference(参考书目)
(大方向书籍,我要是能全部买下来就好了...并没有全部看完,有的只是看过某一章节。Grammar和syntax知乎里面有很多问答跟这方面有关,在此不重复了。)
Cruse, Alan. "Meaning in language: An introduction to semantics and pragmatics." (2011).
Karttunen, Lauri (1974) [1]. Theoretical Linguistics 1 181-94. Also in Pragmatics: A Reader, Steven Davis (ed.), pages 406-415, Oxford University Press, 1991.
Kadmon, Nirit. "Formal pragmatics semantics,pragmatics,presupposition, and focus." (2001).
Levinson, Stephen C. Pragmatics.Cambridge: Cambridge University Press, 1983, pp. 181-184.
Wardhaugh, Ronald. An introduction to sociolinguistics. John Wiley & Sons, 2010. (这本书的影响力很大,有很多跟social science的讨论)
(具体其他上面提到的,每一篇我都仔细读过的)
Monroe, Will, and Christopher Potts. "Learning in the rational speech acts model." arXiv preprint arXiv:1510.06807 (2015).(这篇是关于rsa如何被用于具体task上的)
Farkas, Richárd, et al. "The CoNLL-2010 shared task: learning to detect hedges and their scope in natural language text." Proceedings of the Fourteenth Conference on Computational Natural Language Learning---Shared Task. Association for Computational Linguistics, 2010. (上文提到的hedge and cues shared task,关于linguistics里的现象是如何被formulate成nlp问题的)
Morante, Roser, and Eduardo Blanco. "* SEM 2012 shared task: Resolving the scope and focus of negation." Proceedings of the First Joint Conference on Lexical and Computational Semantics-Volume 1: Proceedings of the main conference and the shared task, and Volume 2: Proceedings of the Sixth International Workshop on Semantic Evaluation. Association for Computational Linguistics, 2012. (negation 的shared task)
最后附上两篇老爷爷对我影响最大的:
Zadeh, Lotfi Asker. "The concept of a linguistic variable and its application to approximate reasoning—I." Information sciences 8.3 (1975): 199-249.
Zadeh, Lotfi A. "The concept of a linguistic variable and its application to approximate reasoning—II." Information sciences 8.4 (1975): 301-357.( 这系列work分两部。)
Zadeh, Lotfi A. "Toward a theory of fuzzy information granulation and its centrality in human reasoning and fuzzy logic." Fuzzy sets and systems 90.2 (1997): 111-127.
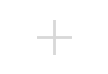